how small differences in data analysis make huge differences in results
- Written by Hannah Fraser, Postdoctoral Researcher , The University of Melbourne
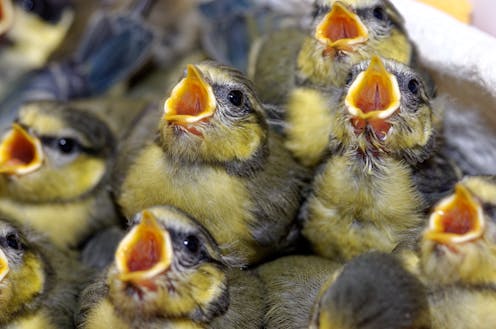
Over the past 20 years or so, there has been growing concern that many results published in scientific journals can’t be reproduced.
Depending on the field of research, studies have found efforts to redo published studies lead to different results in between 23% and 89% of cases.
To understand how different researchers might arrive at different results, we asked hundreds of ecologists and evolutionary biologists to answer two questions by analysing given sets of data. They arrived at a huge range of answers.
Our study has been accepted by BMC Biology as a stage 1 registered report and is currently available as a preprint ahead of peer review for stage 2.
Why is reproducibility a problem?
The causes of problems with reproducibility are common across science. They include an over-reliance on simplistic measures of “statistical significance” rather than nuanced evaluations, the fact journals prefer to publish “exciting” findings, and questionable research practices that make articles more exciting at the expense of transparency and increase the rate of false results in the literature.
Much of the research on reproducibility and ways it can be improved (such as “open science” initiatives) has been slow to spread between different fields of science.
Interest in these ideas has been growing among ecologists, but so far there has been little research evaluating replicability in ecology. One reason for this is the difficulty of disentangling environmental differences from the influence of researchers’ choices.
One way to get at the replicability of ecological research, separate from environmental effects, is to focus on what happens after the data is collected.
Birds and siblings, grass and seedlings
We were inspired by work led by Raphael Silberzahn which asked social scientists to analyse a dataset to determine whether soccer players’ skin tone predicted the number of red cards they received. The study found a wide range of results.
We emulated this approach in ecology and evolutionary biology with an open call to help us answer two research questions:
“To what extent is the growth of nestling blue tits (Cyanistes caeruleus) influenced by competition with siblings?”
“How does grass cover influence Eucalyptus spp. seedling recruitment?” (“Eucalyptus spp. seedling recruitment” means how many seedlings of trees from the genus Eucalyptus there are.)
Two hundred and forty-six ecologists and evolutionary biologists answered our call. Some worked alone and some in teams, producing 137 written descriptions of their overall answer to the research questions (alongside numeric results). These answers varied substantially for both datasets.
Looking at the effect of grass cover on the number of Eucalyptus seedlings, we had 63 responses. Eighteen described a negative effect (more grass means fewer seedlings), 31 described no effect, six teams described a positive effect (more grass means more seedlings), and eight described a mixed effect (some analyses found positive effects and some found negative effects).
For the effect of sibling competition on blue tit growth, we had 74 responses. Sixty-four teams described a negative effect (more competition means slower growth, though only 37 of these teams thought this negative effect was conclusive), five described no effect, and five described a mixed effect.
What the results mean
Perhaps unsurprisingly, we and our coauthors had a range of views on how these results should be interpreted.
We have asked three of our coauthors to comment on what struck them most.
Peter Vesk, who was the source of the Eucalyptus data, said:
Looking at the mean of all the analyses, it makes sense. Grass has essentially a negligible effect on [the number of] eucalypt tree seedlings, compared to the distance from the nearest mother tree. But the range of estimated effects is gobsmacking. It fits with my own experience that lots of small differences in the analysis workflow can add to large variation [in results].
Simon Griffith collected the blue tit data more than 20 years ago, and it was not previously analysed due to the complexity of decisions about the right analytical pathway. He said:
This study demonstrates that there isn’t one answer from any set of data. There are a wide range of different outcomes and understanding the underlying biology needs to account for that diversity.
Meta-researcher Fiona Fidler, who studies research itself, said:
The point of these studies isn’t to scare people or to create a crisis. It is to help build our understanding of heterogeneity and what it means for the practice of science. Through metaresearch projects like this we can develop better intuitions about uncertainty and make better calibrated conclusions from our research.
What should we do about it?
In our view, the results suggest three courses of action for researchers, publishers, funders and the broader science community.
First, we should avoid treating published research as fact. A single scientific article is just one piece of evidence, existing in a broader context of limitations and biases.
The push for “novel” science means studying something that has already been investigated is discouraged, and consequently we inflate the value of individual studies. We need to take a step back and consider each article in context, rather than treating them as the final word on the matter.
Read more: The science 'reproducibility crisis' – and what can be done about it
Second, we should conduct more analyses per article and report all of them. If research depends on what analytic choices are made, it makes sense to present multiple analyses to build a fuller picture of the result.
And third, each study should include a description of how the results depend on data analysis decision. Research publications tend to focus on discussing the ecological implications of their findings, but they should also talk about how different analysis choices influenced the results, and what that means for interpreting the findings.
Authors: Hannah Fraser, Postdoctoral Researcher , The University of Melbourne